How digital analytics can help locate and detect leaks
Currently, the estimated daily personal water consumption rate in the United Kingdom is on average 142 liters per day (L/day) (as of 2021), equating to an estimated total daily usage of 14 billion L/day. This can be compared to the U.S. where on average the daily personal water consumption rate is between 300 – 380 liters, equating to a total daily usage of 1,305 billion liters.
Given that the expected impact of population increase, and climate change are expected to have a negative effect on the amount of freshwater available, we need to develop careful control over the amounts of freshwater we use and lose.
Globally, it was estimated that in 2020 126 billion cubic meters of water was lost due to non-revenue water. This can be compared to England and Wales where an estimated 3.113 billion liters of water are lost through leakage in the delivery networks. Worldwide, climate change is going to have a significant impact on the volume of usable freshwater, and by 2025 it has been estimated that two-thirds of the world’s population may face water shortages.
This increase is predicted to be greater in areas that are already classed as water stressed such as London and the southeast. In addition to this, Environment Agency predictions show that water levels could drop by between 10% and 15% over the next few decades due to climate change. Similar drops are expected around the world representing a growing gap between what the industry can supply and what is required by customers.
Modern Digital Analysis Techniques
The availability of inexpensive computing power and measurement databases has enabled the development of powerful data analysis techniques that allow metering networks to be monitored in real-time. Such techniques can give operators across the globe details about flow meter performance and leakage and are much more effective than the traditional water balance calculation over the distribution network.
TÜV SÜD National Engineering Laboratory has undertaken extensive research into digital analytical techniques to improve the information gathered by modern flow meters. There are two types of computer models used for solving engineering problems: physics-based models and data-driven models. These two classes of computer models differ from the way they represent physical processes.
Physics-based models attempt to gain knowledge and derive decisions through explicit representation of physical rules and generating hypotheses regarding the underlying physical system. Physics-based models driven by physical processes can normally be described by a set of mathematical (theoretical) equations. For example, Navier-Stokes (N-S) equations explain the motion of fluids and can govern Newton’s second law of motion for fluids.
It should be noted that such models do not contain the full complexity of the true physical phenomenon. Instead, they provide a less complex (but valuable) abstraction that approximates the real system. Because these models do not necessarily require knowledge about the physics of the processes, they are very flexible when testing different hypotheses and making predictions.
Data is the Most Valuable Asset
Optimizing data utilization is an operational imperative, especially to water companies under environmental, regulatory and resource pressure. Failure to protect significant metering investments, by not complementing it with modern and cost-effective data analysis techniques, risks increased capital and operational expenditure through poor targeting of effort.
Therefore, smart metering and network analysis will have to be used together to achieve the improvements necessary to meet the challenges facing the water industry on a global scale. This will give water companies much more confidence in their data, alongside their investment decisions and operational expenditure levels. The application of these techniques, along with the recent advances in electronics and computing power, will give water companies the tools to meet the challenges facing them in the 21st century.
We are currently undertaking a project, funded by the U.K. Government’s Department of Science, Innovation and Technology Flow Programme, that aims to investigate if a leak prediction tool can be developed. The aim of the project is to take real field network data provided by water companies and determine if it is possible to use Artificial Intelligence and Machine Learning (AI/ML) techniques to develop a model that can predict when a leakage event is likely to occur.
It is important that the water industry embrace the use of digital analytical tools, together with the use of AI and ML to help develop better network management systems that can be used to help detect and predict water leakage in their networks. If the world is to meet the future demands for freshwater it is vital that global leakage levels are reduced. WW
About the Author
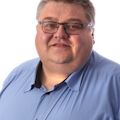
Carl Wordsworth
Carl Wordsworth is Head of Water Sector at TÜV SÜD National Engineering Laboratory, a world-class provider of technical consultancy, research, testing and program management services. Part of the TÜV SÜD Group, it is also a global center of excellence for flow measurement and fluid flow systems and is the UK’s Designated Institute for Flow Measurement